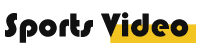
Shot segmentation is an important and challenging task in video understanding. The existing benchmarks either simply focus on shot boundary detection or lack well-defined shot categories for segmentation. In this paper, we present a new fine-grained dataset for shot segmentation as well as shot boundary detection in multiple sports scenes, coined as SportsShot. It consists of 1,200 sports videos, over 4M frames, and over 30K shot annotations. Our SportsShot is characterized with important properties of well-defined shot boundaries, fine-grained shot categories of complexity, and high-quality annotations with consistency, resulting in more challenges in both shot segmentation and boundary detection. In particular, we group the sports shot into seven semantic categories, including close-up, close shot, full view, audience, transition, zooming and others. These semantic categories are of great importance for the subsequent sport activity analysis. We adapt several shot segmentation baseline methods to our SportsShot and conduct error analysis and ablation studies for a better understanding of the key challenges. We hope our dataset can serve as a standard benchmark for shot segmentation and boundary detection in the future.
Our SportsShot dataset focuses on sports scenes, so we search for volleyball, basketball, and football competition videos of levels like Olympics and World Cup on YouTube. These videos are recorded by professional high-definition cameras, guaranteeing excellent video quality. While selecting high quality videos from high-level competitions limits our video sources, our dataset covers complex and diverse shot changes and avoids problems like shaky footage that may cause blurred content. To ensure data balance across different sports, we manually select and cut these official competition records into 400 videos per sport. Each video clip is in high definition, with a minimum resolution of 720p, and a consistent frame rate of 25fps.
For the shot segmentation task, we define 7 shot categories with semantic information: full view; close-up; close shot; audience; transition; zooming; other.
Our SportsShot consists of 1200 videos from 147 competitions of three sports. The original videos are manually selected and cut into 400 videos per sport to keep data balance between sports. Each video is strictly limited to two to three minutes in length.
Since there are few references for the shot segmentation task, we use accuracy and segmental F1-scores to analyze segmentation performance following the standard practice in the temporal action segmentation task, in which accuracy evaluates the predictions in a frame-wise manner, while segmental F1-scores measure the temporal overlap between predicted and ground truth segments at different thresholds. For shot boundary detection, we utilize the precision, recall, and F1 scores, for it is important to detect shot boundaries both precisely and thoroughly.
Please refer to the huggingface page or the competition page to download the dataset for more information.