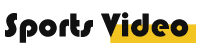
Given natural language queries and video clips, spatio-temporal video grounding task aims to output the most relevant segments and locate the corresponding regions in video clips. However, existing datasets have limited content regarding human actions and do not cover multi-person scenes. In this work, we propose a spatio-temporal video grounding dataset for sports videos, coined as SportsGrounding. We analyze the important components for constructing a realistic and challenging dataset for spatio-temporal video grounding by proposing two criteria: (1) grounding in multi-person scenes and motion-dependent contexts, and (2) well-defined boundaries. Based on these guidelines, we build the SportsGrounding v1.0 dataset by collecting 526 video clips of basketball category and annotating 4,479 instances with 113k bounding boxes. To benchmark this dataset, we adapt several baseline methods and provide an in-depth analysis of the results. We hope that our SportsGrounding will contribute to advancements in future methods in this field.
We provide 526 sports clips in the basketball category, utilizing video data from the MultiSports dataset, which are collected from the Olympic Games, NCAA Championship, and NBA on YouTube. We merge adjacent annotated instances of the same subject and provide corresponding natural language descriptions. These descriptions are unique throughout the video clip and clearly describe the visual attributes of the subjects (such as clothing, position, etc.), actions, and relationships, while also encouraging the interaction descriptions involving other individuals and relevant subjects on the court.
The SportsGrounding dataset retains the uncut original long video data, with the number of complete videos associated with the annotated instances comparable to that of the HC-STVG dataset. Both the HC-STVG and SportsGrounding datasets have the longest average description lengths, with relatively rich content. In addition to appearance and actions, the text descriptions in the SportsGrounding dataset also include interaction information between the subjects and other players, which places higher demands on the model's scene modeling capabilities.
The duration of instances in the SportsGrounding dataset ranges from approximately 0.2 seconds to over 16 seconds. Compared to the VidSTG and HC-STVG datasets, the duration differences between annotated instances in the SportsGrounding dataset are more pronounced, with generally shorter segment durations, which presents a greater challenge for spatio-temporal grounding.
We employ the m_tIoU, m_sIoU and vIoU@R as evaluation criteria. The m_tIoU is the average temporal IoU between the selected clips and ground truth clips, the m_sloU is the average spatial IoU within the true start and end frames and voU@R is the proportion of samples which vloU > R.