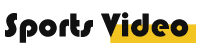
Video-based visual relation detection tasks, such as video scene graph generation, play important roles in fine-grained video understanding. However, current video visual relation detection datasets have two main limitations that hinder the progress of research in this area. First, they do not explore complex human-human interactions in multi-person scenarios. Second, the relation types of existing datasets have relatively low-level semantics and can be often recognized by appearance or simple prior information, without the need for detailed spatio-temporal context reasoning. Nevertheless, comprehending high-level interactions between humans is crucial for understanding complex multi-person videos, such as sports and surveillance videos. To address this issue, we propose a new video visual relation detection task: video human-human interaction detection, and build a dataset named SportsHHI for it. SportsHHI contains 34 high-level interaction classes from basketball and volleyball sports. 118,075 human bounding boxes and 50,649 interaction instances are annotated on 11,398 keyframes. To benchmark this, we propose a two-stage baseline method and conduct extensive experiments to reveal the key factors for a successful human-human interaction detector. We hope that SportsHHI can stimulate research on human interaction understanding in videos and promote the development of spatio-temporal context modeling techniques in video visual relation detection.
Please choose "1080P" for better experience.
We focus on high level interactions between athletes, which are semantically complex and require detailed spatio-temporal context reasoning to recognize. With the guidance of professional athletes, we generated the final interaction vocabulary through iterative trial labeling and modification.
Following common practice in AVA and AG datasets, we define interaction instances at the frame level, with reference to a long-term spatial-temporal context. Each interaction instance can be formulated as a triplet ⟨S,I,O⟩ where S and O denote the bounding boxes of the subject and object person and I denotes the interaction category between them from the in teraction vocabulary. When the subject person or the object person is out of view, we annotate S or O as “invisible”. This happens infrequently and we will provide statistics about it in the appendix.
We carefully selected 80 basketball and 80 volleyball videos from the MultiSports dataset to cover various types of games including men’s, women’s, national team, and club games. The average length of the videos is 603 frames and the frame rate of the videos is 25FPS. All videos have a high resolution of 720P.
OurSportsHHI provides interaction instance annotations on keyframes of basketball and volleyball videos. As shown in Table 1, SportHHI contains 34 interaction classes, and 11398 keyframes in total are annotated with instances. Current video scene graph datasets deal with general relations between various kinds of objects while our SportsHHI focuses on high-level interaction between humans. It is reasonable that datasets for video scene graph generation have a larger scale than SportsHHI. However, our SportHHI still has a comparable size to the popular VidVRD dataset for video scene graph generation. Our SportsHHI has more annotated keyframes (11398 versus 5834) and the number of interaction instances is close (55631 versus 50649). One important characteristic of our SportsHHI is the multi-person scenarios. The average number of people per frame in our SportsHHI is much higher than AG andVidVRD. AG only contains one person in each video and there is virtually no multi-person scenario in videos of VidVRD. Human-human interaction is barely involved in these datasets.
A prediction is considered as a true positive if and only if its subject and object bounding boxes both have an IoU overlap higher than a preset threshold with their counterparts in a ground-truth interaction instance and the predicted interaction class matches the ground truth. Following VidVRD, we set the IoU threshold to 0.5. Following the video scene graph generation task, we use Recall@K(K is the number of predictions) as the evaluation metric. Mean average precision is adopted as an evaluation metric for many detection tasks. However, this metric is discarded by many former visual relation detection benchmarks because of their incomplete annotation. This issue does not exist in SportsHHI. In our experiments, mAP is also reported for the interaction detection task. We argue that mAP is a more difficult and informative metric. Two different modes for model training and evaluation are used: 1)human-human interaction detection (HHIDet) which expects input video frames and predicts human boxes and interaction labels; 2) human-human interaction classification(HHICls) which directly uses gound-truth human bounding boxes and predicts human-human interaction classes.
Please refer to the huggingface page or the competition page to download the dataset for more information.