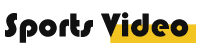
Multi-object tracking in sports scenes plays a critical role in gathering players statistics, supporting further analysis, such as automatic tactical analysis. Yet existing MOT benchmarks cast little attention on the domain, limiting its development. In this work, we present a new large-scale multi-object tracking dataset in diverse sports scenes, coined as SportsMOT, where all players on the court are supposed to be tracked. It consists of 240 video sequences, over 150K frames (almost 15× MOT17) and over 1.6M bounding boxes (3× MOT17) collected from 3 sports categories, including basketball, volleyball and football. Our dataset is characterized with two key properties: 1) fast and variable-speed motion and 2) similar yet distinguishable appearance. We expect SportsMOT to encourage the MOT trackers to promote in both motion-based association and appearance-based association. We benchmark several state-of-the-art trackers and reveal the key challenge of SportsMOT lies in object association. To alleviate the issue, we further propose a new multi-object tracking framework, termed as MixSort, introducing a MixFormer-like structure as an auxiliary association model to prevailing tracking-by-detection trackers. By integrating the customized appearance-based association with the original motion-based association, MixSort achieves state-of-the-art performance on SportsMOT and MOT17. Based on MixSort, we give an in-depth analysis and provide some profound insights into SportsMOT.
Please choose "1080P" for better experience.
We provide 240 sports video clips of 3 categories (i.e., basketball, football and volleyball), where are collected from Olympic Games, NCAA Championship, and NBA on YouTube. Only the search results with 720P resolution, 25 FPS, and official recording are downloaded. All of the selected videos are cut into clips of average 485 frames manually, in which there is no shot change.
As for the diversity of video context, football games provide outdoor scenes and the rest results provide indoor scenes. Furthermore, the views of the playing courts do vary, which include common side view of crowded audience like in NBA, views from the serve zone in volleyball games, and aerial view in football games. Diverse scenes in our dataset will encourage the algorithms to generalize to different sports tracking settings.
There are a few examples as follows.
v_iIxMOsCGH58_c013
v_4LXTUim5anY_c013
v_2Dnx8BpgUEs_c007
There are 240 clips of the average 495 frames(19.8 seconds) in SportsMOT. We manually divide them into training, validation and test set, containing 45, 45, 150 videos respectively. It's guaranteed that every split does not have video clips from the same game.
To measure the motion patterns of quantitatively, we introduce fragment speed.
We regard a track of identical ID, one start and one end point as a fragments. The speed of a fragment is the sum of center displacement between every 2 frames.
And we use deformation rate to measure the degree of deformation. Here, wmin hmin refer to the minimum width and height of bounding boxes in a track fragment.
• Other tracking datasets (e.g., MOT20/) used for pretraining are forbidden.
• Each team can have one or more members.
For our benchmark and challenge, we consider HOTA as the main metric. More specifically, this metric can be decomposed into two components: DetA and AssA, focusing on detection and association accuracy, respectively.
Please refer to the huggingface page or the competition page to download the dataset for more information.